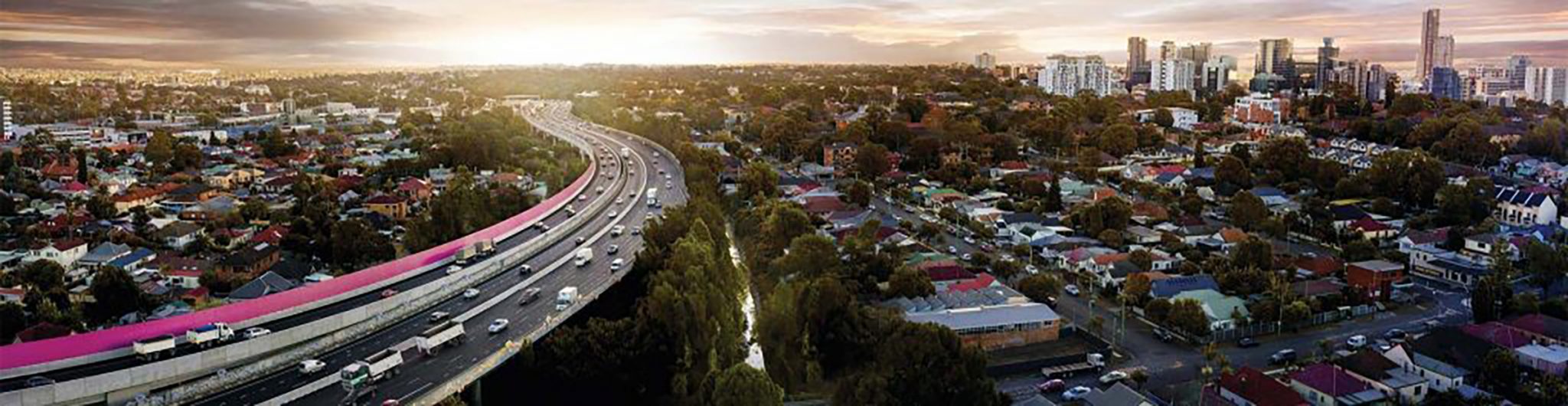
STM Re-estimation
Transport for New South Wales commissioned VLC for a comprehensive improvement program for Greater Sydney’s main strategic transport model, TfNSW’s Strategic Transport Model (STM).
Client requirements
The comprehensive improvement program commissioned by TfNSW included:
- Rebasing the model to 2016
- Streamlining the Population Synthesiser module
- Doubly-constraining home-work trip ends
- Including the land-use density variables to predict mode choice
- Addressing base year calibration and pivoting issues
- Allowing car-ownership to be user-definable
- Introducing public transport crowding
- Producing standard economic outputs
Delivered outcome
Rebasing the model to 2016
VLC rebased the model to 2016, using updated HTS and Census information to re-estimate and recalibrate the mode-destination choice models. VLC developed standardised model estimation and validation Jupyter notebooks, using the Larch Python module, allowing TfNSW to run these notebooks themselves. Land-use density variables were included in the estimation of these models, removing the need for destination-specific constants and improving forecast ability of the STM. RAND also re-estimated the tour frequency, licence holding and car ownership models, which VLC integrated into the STM. VLC also developed public transport validation processes, using Opal tap-on/off data at the SA2/SA3 level.
Population Synthesiser
VLC re-developed the Population Synthesiser process using the Python-based open-source PopSim module. These processes were set up using Jupyter notebooks. This is a transparent and comprehensive process including validation outputs, improving accuracy of demographic forecasts by allowing TfNSW to generate future synthetic populations themselves to reflect different demographic inputs, rather than applying adjustment factors. The Population Synthesiser module was also improved to match demographic targets at the zonal level, compared to regional levels previously.
Additional model capabilities
VLC also added the following model capabilities:
- Integration of the licence holding and car ownership models into the STM. This included allowing the user to define car ownership levels.
- Doubly-constraining home-work trip ends, improving model fit without use of destination-specific constants
- Introducing public transport crowding
- Producing standard economic outputs as part of an STM model run